Extraction de METAdonnées par REconnaissance VocalE
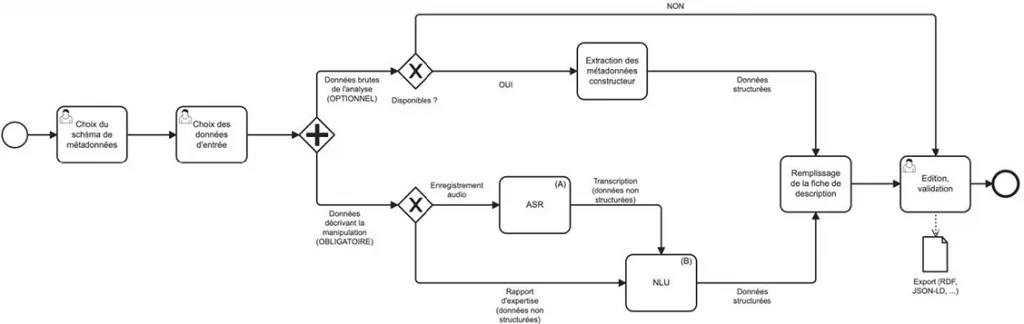
For the heritage sciences, a field for the production of societal and collective knowledge based on a perpetual confrontation between material objects and multi-disciplinary studies combining human and social sciences and experimental sciences, the challenges of digital data management are today, thanks to the acquisition of recent maturity, confronted with the strong heterogeneity of documentary sources (texts, images, videos, etc.), analytical data (from various sensors, imaging techniques, sample analyses, etc.) and processing processes mobilised for the purposes of description, analysis, monitoring or conservation. ), analytical data (from a variety of sensors, imaging techniques, sample analysis, etc.) and processing methods used for description, analysis, monitoring and conservation. Metadata and provenance paradata are the guarantors of the reliability of data, providing invaluable information about the contexts in which it was acquired and the possibilities for subsequent re-use. However, their rigorous production is often time-consuming and even tedious, as the information to be provided is both diverse and difficult to retrieve at a later date. In this context, the interdisciplinary METAREVE project proposes to simplify this process by accompanying it from the production of data in the field to its ex situ processing. The innovative method proposed aims to automate the extraction of this information using automatic speech understanding approaches borrowed from the field of artificial intelligence, drawing on thesauri constructed by the various communities involved in heritage science. In particular, it aims to provide a software building block that can feed the ecosystem developed as part of the EquipEx+ ESPADON.
Contact : Violette Abergel
MAP members : Violette Abergel
Project partners : Vincent Detalle (SATIE), Olivier Malavergne (CRC), Besma Zeddini (SATIE)
Funding : 2023-2024 (Fondation des Sciences du Patrimoine)
References:
[1] Ministère de la Culture et de la Communication – Secrétariat général, « Métadonnées culturelles et transition Web 3.0. », Ministère de la Culture et de la Communication, Paris, France, Feuille de route stratégique 2014‑01, 2014.
[2] M. D. Wilkinson et al., « The FAIR Guiding Principles for scientific data management and stewardship », Sci Data, vol. 3, no 1, p. 160018, 2016, doi: 10.1038/sdata.2016.18.
[3] B. Mons, et al., « Cloudy, increasingly FAIR; revisiting the FAIR Data guiding principles for the European Open Science Cloud », ISU, vol. 37, no 1, p. 49‑56, 2017, doi: 10.3233/ISU-170824.
[4] L. Castelli, et al., « Heritage Science and Cultural Heritage: standards and tools for establishing cross-domain data interoperability », Int J Digit Libr, vol. 22, no 3, p. 279‑287, sept. 2021, doi: 10.1007/s00799-019-00275-2.
[5] D. Myers, et al., « The Arches heritage inventory and management system: a platform for the heritage field », Journal of Cultural Heritage Management and Sustainable Development, vol. 6, no 2, p. 213‑224, 2016, doi: 10.1108/JCHMSD-02-2016-0010.
[6] S. Tournon, et al., « Comment gérer les projets 3D collaboratifs en SHS? », in Humanistica 2020, Bordeaux, France, mai 2020, 11p.
[7] J. Li, et al., « A survey on deep learning for named entity recognition », IEEE Transactions on Knowledge and Data Engineering, vol. 34, no 1, p. 50‑70, 2020.
[8] S. Van Hooland, et al., « Named-entity recognition: a gateway drug for cultural heritage collections to the linked data cloud ? », Literary and Linguistic Computing, vol. 1, 2013.
[9] S. Van Hooland, et al., « Exploring entity recognition and disambiguation for cultural heritage collections », Digital Scholarship in the Humanities, vol. 30, no 2, p. 262‑279, 2015, doi: 10.1093/llc/fqt067.
[10] A. Felicetti, et al., « NLP Tools for Knowledge Extraction from Italian Archaeological Free Text », in 2018 3rd Digital Heritage International Congress (DigitalHERITAGE), San Francisco, CA, USA: IEEE, 2018, p. 1‑8. doi: 10.1109/DigitalHeritage.2018.8810001.
[11] M. Ehrmann, et al., « Named Entity Recognition and Classification on Historical Documents: A Survey », 2021, doi: 10.48550/ARXIV.2109.11406.
[12] A. Bombini, et al., « A Cloud-Native Web Application for Assisted Metadata Generation and Retrieval: THESPIAN-NER », Applied Sciences, vol. 12, no 24, 2022, doi: 10.3390/app122412910.
[13] M. Malik, et al., « Automatic speech recognition: a survey », Multimedia Tools and Applications, vol. 80, p. 9411‑9457, 2021.
[14] W3C, « Web Speech API, draft community group report », 18 août 2020. https://wicg.github.io/speech-api/ (consulté le 31 mars 2023).
[15] J.-M. Vallet et al., « Development of a NDT toolbox dedicated to the conservation of wall paintings: Application to the frescoes chapel in the Charterhouse of Villeneuve-lez-Avignon (France) », in 2013 Digital Heritage International Congress (DigitalHeritage), Marseille, France: IEEE, 2013, p. 67‑74. doi: 10.1109/DigitalHeritage.2013.6744731.